Proteomics Approach Working Its Way To The Clinic For Stubborn Cancers
By Deborah Borfitz
April 2, 2024 | For roughly half of patients with recurrence of often-deadly glioblastoma multiforme (GBM), their tumors are characterized by cells that look a lot like healthy neurons. The mimicry trick was discovered by proteomics, which has opened a view to tumor alterations unavailable through genetics alone, according to Antonio Iavarone, M.D., professor of neurological surgery and deputy director of the Sylvester Comprehensive Cancer Center at the University of Miami Miller School of Medicine.
Moreover, it appears possible to delay or treat these neuronal recurrent glioblastomas by targeting their most active and important “master kinases,” he adds. This hope arises from a newly completed study, appearing in Cancer Cell (DOI: 10.1016/j.ccell.2023.12.015). It was the first time proteomics has been used to examine glioblastoma’s transition from treatable to treatment-resistant.
Master kinases can be identified for every single tumor and patient and disease stage, says Iavarone, and on that basis drugs can be selected for testing on tumor avatars (organoids) grown in the lab from patient-derived cells collected from the same tumor sample on which molecular analysis is separately done. In the latest study, researchers tested an inhibitor of the BRAF (B-raf proto-oncogene) kinase on patient avatars to impair both neuronal transition and migration capability of recurrent tumor cells. When coupled with chemotherapy (temozolomide), the BRAF inhibitor (vemurafenib) significantly extended survival of the mice harboring human tumor organoids in their brain.
Discussions are now underway about a clinical trial that would test BRAF inhibition in patients whose disease is in the neuronal state, one of four subtypes of GBM identified by Iavarone and his team (Nature Cancer, DOI: 10.1038/s43018-020-00159-4). They will be looking at other BRAF inhibitors to be sure they choose the one that works most efficiently at preventing cancer from transitioning to the resistant state, he adds.
Marketed BRAF inhibitors, including vemurafenib, primarily target mutated versions of the BRAF protein, but that rarely occurs in glioblastoma, Iavarone points out. Investigators will therefore be looking carefully at inhibitors of the unmutated, wild type of BRAF.
Improving Predictions
Proteomics is now used extensively in the research setting to study cancer, but to date has had “limited impact in the clinical decision-making process,” says Iavarone. No proteomics-based clinical test exists to evaluate selection of patients for a particular type of therapy or stratification of participants in clinical trials.
But that is the promise, he says, and the latest study provides ample opportunity for the realization of clinical proteomics. The evolutionary trajectory of GBM was essentially a mystery before now.
The vast majority of GBM patients receive a genetic test on their tumor that identifies the somatic mutated genes that might be addressed therapeutically. But the treatments chosen are often ineffective, with therapeutic failure being the norm rather than the exception, says Iavarone.
Relative to what is happening in research labs, genetic testing is a primitive way to analyze tumors. Iavarone and his colleagues assembled what became the largest dataset of its kind featuring matched tumor samples from 123 glioblastoma patients both at diagnosis and then recurrence after initial therapy. This is how they learned that the tumors early on primarily featured the ability to proliferate but later tended to transition to a neuronal state to survive therapy.
By studying the proteomes and protein modifications in the samples, they were able to detect important changes not previously seen in similar cancer studies examining the genomes or transcriptomes of tumors. The key takeaway here is that “the more understanding we generate on individual tumors, the more accurate are the therapeutic predictions,” says Iavarone.
Creation of the avatars to test best-treatment predictions is a process that can be completed within a month in the right setting, he adds. That aligns well with the wait time between when surgery happens and when patients are sufficiently healed to receive drugs. In addition to in vitro research models, drug testing can also happen after transplantation of the tumor cells into the mouse brain.
Generating the required data is the major challenge because of the cost and effort involved, and generation of the avatars for drug testing adds another layer of complexity, Iavarone says. Identification of master kinases, which happens with the aid of machine learning, would need to meet the regulatory bar of the Food and Drug Administration to be used for medical purposes. So, too, would any kinase-inhibiting drug that is on the marketing track.
Master Kinases
Key learnings were achieved by Iavarone and his team using proteomic and phosphoproteomic data, the latter concerning a modification (phosphorylation) by which proteins get activated. Computational analysis on the proteomics dataset allowed them to identify the most active kinases in the recurrent cancers—an exercise that could be done for any tumor where proteomics data are available, Iavarone says.
An article about the tumor classification algorithm was published last year in Nature Cancer (DOI: 10.1038/s43018-022-00510-x). In this case, it was used in conjunction with integrative multi-omics to qualify the master kinases of the glycolytic/plurimetabolic and proliferative/progenitor subtypes of GBM to aid the identification of actionable therapeutic targets.
The machine learning algorithm has also been implemented for other tumor types, including breast and lung cancers. “Kinases are the most important enzymes in cancer in terms of their targetability, because there are many drugs that can inhibit [them].” Given a tumor avatar, kinase predictions can be tested to ensure the treatment ultimately chosen for an individual patient is the one that will deliver the most promising therapeutic effect.
Researchers separately hope to secure NCI funding to pursue testing of a drug identified by the algorithm as being promising for the treatment of patients with the mitochondrial subtype of GBM. This subtype is associated with the most favorable clinical outcome, which Iavarone and his team recently found was markedly vulnerable to inhibitors of oxidative phosphorylation—a list that includes metformin.
Given unlimited financial resources, it is now possible to study even the most aggressive and treatment-resistant tumors and come up with therapeutic opportunities, he notes. But money isn’t the only hurdle. Clinicians want easy solutions to this complex problem, and regulatory science around proteomics is still lacking.
At the University of Miami, a Transformational Cancer Research Building is now going up that is devoted to the idea of accelerating the translation of groundbreaking scientific discoveries and fully personalizing patient care. It may be one of the first places a few years from now where Iavarone’s research-based work will be implemented into the clinical setting.
Leave a comment
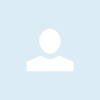
