Discussions Begin On Properly Using Generative AI In Clinical Trials
By Deborah Borfitz
March 19, 2024 | The question of whether generative artificial intelligence (AI) can play a role in clinical trials needs to be supplanted by questions of where and when it should be applied, according to a panel of experts speaking at last month’s Summit for Clinical Ops Executives (SCOPE) in Orlando. The focus is of necessity shifting to issues of “security, privacy, ethics, compliance, and value, and that’s the governance framework that is missing... [and has] regulators scrambling a little bit,” according to moderator Brian Martin, head of AI in information research and a research fellow at AbbVie.
The “charge and the challenge,” he says, is to not get so enamored of technology as to lose sight of the fact that the end goal is improving patient care. To that end, SCOPE producers plan to promote ongoing conversation on the AI topic via a series of quarterly webinars this year.
As it is, AI occupies a tiny portion of the precision health space and ideally serves as a catalyst of change although it is “far from perfect,” says Hoifung Poon, general manager at Health Futures of Microsoft Research and an affiliated professor at the University of Washington Medical School. Even GPT-4, the latest version of the popular large language model that generates text from textual and visual inputs, can make hallucination and omission errors.
The symbiotic relationship between humans and computers is empowering experts to become “super-human,” curating patient notes in minutes rather than hours and dramatically improving operational cycle time performance on clinical studies, he continues. “Generative AI disruption is enabling us for the first time ever to start imagining high-fidelity patient embedding from real-world data that can almost serve as a digital twin for the patient.” Potential capabilities include “predicting the next major medical event, like disease progression and tumor response.”
Tangible progress can already be seen with the “universal structuring capability” of GPT-4, says Poon, which has enabled efficient abstraction of patient information from “noisy, unstructured” clinical text at a large scale. Intriguingly, GPT-4 can also “self-check” to fix its own hallucinations and omission errors. He says he is most excited by the capability of multimodal generative AI systems to accept multiple types of inputs, including imaging and multiomics, to produce various forms of output.
Productivity Gains
For Pfizer, the value of AI tools has evolved over time, says Prasanna Rao, head of AI and data science for data management and monitoring. Among the first use cases was automating medical coding using “transformer generation” models. That was followed by models for cleaning clinical trial data of anomalies.
The company has more recently invested in knowledge graphs that in the next generation will be combined with large language models for different use cases in clinical development by making more data visible at once in real time, Rao says. Pfizer is also actively using Microsoft Copilot to achieve productivity gains, to for example simplify protocol authoring.
Unlike in the past, when AI was an “all or none event,” humans and machines now work in a much more hybrid environment, says Neil Garrett, head of regulatory medical writing at Johnson & Johnson. A huge number of AI tools exist to help evaluate patient burdens, understand feasibility, and inform protocol design, but at the end of the day decisions are still being made by people.
In the realm of medical writing, “the greatest opportunity may be to produce the first draft of a document, whether it’s a protocol or a study report,” Garrett says. Human interpretation is needed in that context—at least for now.
Many people in the medical writing community were initially skeptical of AI’s role in the protocol-writing process, he adds. “My personal opinion is that it is actually there to help us do things faster... better... [and] more efficiently.” AI is going to remove “less interesting” components of the work so human experts can focus on more strategic elements.
Ethical Considerations
The latest large language models can process very large volumes of data as well as understand how variables connect with each other, improving the predictive capabilities of AI, says Samar Noor, vice president and head of statistical programming, global biometric sciences, for Bristol Myers Squibb. “We can also use AI to build new models to really take out the bias.”
AI can help people tailor and understand patient characteristics and outcomes study by study, she continues, but caution is warranted since all models are built on the data collected that isn’t necessarily of high quality and free of bias. Historically, clinical trials were focused primarily on the U.S., and this is also where claims data from electronic health records originate. Europe is catching up, but relatively little data is available from Asia.
There are also ethical considerations, she notes. “Privacy laws... are there for a reason.”
Precision Health
One of the big dreams with AI is to digitize the billions of data points that get generated every day to create real-world evidence at the population scale to empower precision health, says Poon. Of course, establishing causality is a lot harder than showing a correlation because it requires experiments with both controlled and independent variables where results are certain and predictable.
The first step is to use generative AI to help clean data of errors and “non-random missingness,” he says. Given reasonably high-quality, real-world data, it makes sense to start incorporating standard tools such as propensity matching to balance the distributions of measured confounding factors between exposed and unexposed individuals.
Exciting possibilities for the future include using real-world data to do more than what is normally done in the context of a clinical trial, to for example look over longer time horizons, Poon says, or simply to create synthetic control arms more routinely. Eventually, trials could also more often be adaptively designed to ensure no promising treatment arm is missed while randomizing more participants to the better treatments.
Leave a comment
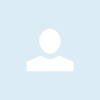
