Five Health Systems Picking Dandelion Health To Advance Clinical AI
By Deborah Borfitz
March 6, 2024 | If artificial intelligence (AI) is to be as insightful as possible in advancing human health, it is not enough to have a patchwork of out-of-context data from which to draw conclusions. That is unfortunately the current situation and at the cost of lost opportunities to better treat patients, identify early biomarkers of disease, and recognize the right endpoints in clinical trials—not to mention eradicating existing biases against marginalized groups of people.
Addressing these glaring gaps is the end game of 4-year-old startup Dandelion Health, which has a large-scale project underway to start connecting healthcare data to patient outcomes. The means, according to CEO Elliott Green, is a high-fidelity, real-world product development platform being built in collaboration with five major health systems across the country. Its output, he says, is clinical data that for the first time includes a wide array of information such as imaging scans, waveforms, and labs all linked to the electronic medical record.
Three health systems— Sharp HealthCare (San Diego, California), Sanford Health (Sioux Falls, South Dakota), and Texas Health Resources (Arlington, Texas)—are already on board with two further systems to be added to the mix this year, which should collectively represent between 15 and 20 million patient records, according to Green. The company has built data pipelines that ensure frequent data refreshes of de-identified data.
In return, the health systems collect a share of the revenue Dandelion Health receives from leasing of the data pool to clients that are expected to include pharmaceutical and biotechnology companies as well as anyone working in the digital health space. The company currently has customers for validating an AI algorithm for predicting heart failure, conducting health economics and outcomes research on a CT algorithm for detecting lung nodules, and developing algorithms that predict ejection fraction from electrocardiograms (ECGs).
Role With GLP-1s
Dandelion Health most recently launched a metabolic data library that supports insights on glucagon-like peptide 1 (GLP-1) and, in conjunction, will soon begin a collaborative research study with another startup focused on analyzing how GLP-1 drugs affect cardiac risk. One of the biggest drug classes in the world, GLP-1 agonists are used to treat type 2 diabetes and not only improve blood sugar control but may also lead to weight loss.
Despite an explosion of interest in GLP-1 drugs—with Eli Lilly (Mounjaro and Zepbound) and Novo Nordisk's (Ozempic) dominating in recent years, and Merck working on a GLP-1 drug for non-alcoholic steatohepatitis (NASH)—"no one really knows that much” about their impact on patients or what other factors are at play affecting patient response to treatment, Green says. All three companies are also now looking to show that their GLP-1 drugs have cardiovascular benefits.
Green’s vision is for real-world patients on each of these drugs to be followed over the next two to four years to see what happens to their cardiac risk scores, and how that relates to their body mass index or other comorbidities. Perhaps other medicines will surface that do a comparable or better job, at least for select patient groups.
Using the Dandelion platform, those possibilities could be explored by tapping into the connected healthcare data of hundreds of thousands of patients taking GLP-1s, says Green. NASH researchers might also decide to look for fat deposits in the liver that incidentally show up on MRI, CT, or ultrasound scans taken for unrelated concerns. The company’s data pool includes hundreds of thousands of echocardiograms and more than three million ECGs that might enable earlier detection and diagnosis of problems, he notes.
All these findings could reveal the relative importance of potential endpoints used in clinical trials and the best inclusion and exclusion criteria for ensuring study success, he adds.
The first big win Green and his team are pursuing is an algorithm that can “run in the wild” to make a meaningful difference. Dandelion Health is effectively setting itself up as a neutral third party where the effectiveness of AI models to, say, spot a lung nodule on a CT scan earlier than the human eye, can be recognized and confidently implemented to improve patient outcomes. This will also be key in terms of health equity, since the dataset features such a broad range of races, ethnicities, and care practice patterns, he adds.
The overarching goal is to increase the efficacy and accuracy of AI models used in healthcare. It is one of few occasions where a for-profit company is “completely aligned” with that outcome, says Green. “The products that people use will be better, and everyone wins.”
‘Complete Greenfield’
Green started his healthcare career at New York-based insurance company Oscar Health, which is when he met Niyum Gandhi, a former Mount Sinai Health System executive and current CFO and treasurer for Mass General Brigham. He and Gandhi are two of the cofounders of Dandelion Health and in 2020 were both being approached by AI-based radiology companies looking to get their hands on data from healthcare systems.
They were subsequently introduced to Sendhil Mullainathan (professor of computational and behavioral science at the University of Chicago) and Ziad Obermeyer, M.D. (associate professor of health policy and management at the University of California, Berkeley), who were to become Dandelion’s two other cofounders. Obermeyer and Mullainathan famously co-authored a research paper on bias in healthcare algorithms (Science, DOI: 10.1126/science.aax2342), which inspired the launch of Dandelion. The study focused on the widely deployed cost prediction algorithm developed by Optum, a division of UnitedHealth Group.
What the company cofounders saw was that despite AI being one of the highest-potential technologies in healthcare, no one had a good implementation plan in terms of making it trustworthy and getting paid for algorithms put to clinical use. “That’s when we realized the issue was ... [that] people just don’t have access to the right data,” says Green, a situation he likens to asking a doctor to make a treatment decision based solely on a patient’s CT results, physician notes, or electronic medical record.
“This is a complete greenfield,” Green says. “Though many are looking at advancement in imaging algorithms, we haven’t had the datasets available at scale to enable us to develop solutions at scale.”
But that won’t happen without the kind of data that can reliably train models, meaning it is “diverse enough that it recognizes everyone,” he says. The data to be contributed by the five health systems in the Dandelion network is “as good an approximation as we can get for the U.S. population as a whole,” in addition to having enormous depth and breadth.
Building Trust
Since all relevant patient data are housed within health systems, and their input is key to building trust in AI algorithms, Dandelion Health decided to make them establishing partners in the startup. “We very much wanted to make sure this was done with clinicians,” says Green. “We don’t believe they are going to be replaced [by AI]; we believe it’s an augmentation, and we would like to see the money generated and the benefits generated going to back to the patients and the healthcare systems.”
Deidentified patient data doesn’t leave Dandelion’s cloud-based development environment, he adds. “Everything is very carefully done and orchestrated, and hospital systems have a lot of say, and so what we have is probably the broadest and deepest and highest fidelity dataset that you can get.”
Some datasets are enabled by joining together multiple sources of data to create an overview of a patient's journey, which can make it difficult to decipher the subtleties of that chain of events, explains Green. Having a single data source across the whole journey, as in the case of Dandelion, can make it “easier to understand the nuances” of decision-making—for example, patient discharge that might variably refer to patient movement from the emergency room to an inpatient ward or full discharge from the hospital. In such instances, “Dandelion can find out from its consortium partners what type of discharge the data corresponds to,” enhancing data quality.
“It’s very hard to make sure you have a high level of fidelity across all that data with so many different people making decisions about how to curate it,” he says. “We feel this is too important a problem and too important an outcome for that to be the case so we centralize everything... we talk to the health systems and spend a lot of time with their analytics teams and with their physicians to make sure that what we have is as close to perfect as we can manage.”
The deidentification process makes it possible to follow the trajectory of a disease in unnamed individuals based on their encounters with the healthcare system where various scans, blood draws, and other tests are performed, says Green. In the same way, cohorts of deidentified patients with the same disease can be studied to look for early biomarkers of the condition and what the objectives of a clinical trial might best be. For NASH, perhaps there are alternatives to expensive and hard-to-do MRI-based liver imaging being done today.
“There is so much data and as humans... we can’t possibly analyze all of it,” Green says. “But a machine can.” Algorithmic techniques themselves haven’t changed much over the last 30 years, he adds, because it is the training data that has needed an upgrade to better speak ground truth.
Leave a comment
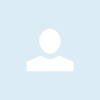
