AI-Based Platform For Detecting AFib Put To The Test In VA Facilities
By Deborah Borfitz
January 23, 2024 | Investigators at Cedars-Sinai Medical Center are looking to minimize the biases in the data used by artificial intelligence (AI) algorithms, most recently a deep learning model trained on nearly a million electrocardiograms (ECGs) to identify people with abnormal heart rhythms. The inputs came from a pair of large Veterans Affairs (VA) hospital networks serving a highly diverse set of patients in terms of demographics and comorbidities.
The goal here is a clinical-grade tool for detecting atrial fibrillation (AFib) in individuals who are not yet showing symptoms, which describes one out of every three people with the condition, according to David Ouyang, M.D., a cardiologist in the Smidt Heart Institute at Cedars-Sinai and a researcher in the division of artificial intelligence in medicine. AFib can lead to blood clots in the heart and increases the risk of stroke, heart failure and other heart-related complications.
In a prognostic study recently published in JAMA Cardiology (DOI: 10.1001/jamacardio.2023.3701), the algorithm proved highly accurate in predicting AFib within 31 days from the deidentified medical records of 980,341 ECGs outpatients with 12-lead ECGs in sinus rhythm served by Cedars-Sinai and six VA hospital networks. The model’s utility is in detecting patterns in ECG readings that are so subtle as to escape detection even by the most highly trained cardiologists, Ouyang says.
It was one of a series of algorithms under development at Cedars-Sinai, including an AI assistant integrated with the institution’s picture archiving and communication system that has been trained to read echocardiograms better than sonographers. Researchers hope to obtain approval from the Food and Drug Administration (FDA) to market the AI cardiac function assessment software, which they seek to make openly available to all.
The main danger with many existing AI algorithms for diagnostic and screening purposes is that they may work only in select populations because they were only evaluated in a single, often affluent academic center—not in heterogenous populations such as military veterans inclusive of minority groups and people with different co-occurring health conditions who may also have less access to healthcare, says Ouyang. For many veterans, the VA hospital system is primarily a surrogate for commercial healthcare that can come with longer wait times and poorer access to care in rural areas.
Over the past few years, the FDA has increasingly expressed its interest in ensuring diversity in the data used to train AI algorithms. Some FDA-approved software has been training on 1,000 or fewer examples, he says.
Most recently, the FDA approved an AI-based platform developed at the Mayo Clinic for the detection of low ejection fraction in patients at risk of heart failure. The algorithm was trained on more than 100,000 ECG and echocardiogram data pairs and clinically tested in over 40,000 patients around the world. It is one of hundreds of devices enabled by AI and machine learning to be authorized by the agency since 1995, of which only a handful (9%) have been for cardiovascular purposes.
The ECG-AI LEF device, marketed by Anumana, was authorized through the FDA’s 510(k) process. Cedar-Sinai’s AFib detection algorithm is a similar use case, says Ouyang, adding that extensive testing of the research algorithm in clinical trials is planned.
Prospective Screening
The chief difficulty in diagnosing AFib is not only that it can be asymptomatic, but that the symptoms can be intermittent and not necessarily appearing when patients are visiting their doctor, Ouyang says. This is especially true for patients who are underserved or might see a cardiologist less often than individuals who are wealthy or access-privileged by virtue of geography, a group that includes many veterans.
AFib isn’t usually life-threatening, he adds, but it does require timely and proper treatment. “In clinical practice, when patients are diagnosed with atrial fibrillation, they also will be started on a blood thinner because there is a risk of stroke.” If detected prior to a non-elective surgery, they might also be more closely monitored because of their higher risk of perioperative complications.
The fact that the algorithm was found to work in diverse settings and patient populations “shows there is a viable path to getting this into broad clinical use,” Ouyang says. Ultimately, the platform may need to be brought to market by a company with commercial interests and the wherewithal to scale its deployment to clinics beyond Cedars-Sinai. The code for implementing the algorithm is publicly available on the online GitHub repository.
Next steps include a planning for a prospective, blinded clinical trial where the algorithm will be used to screen patients for AFib at different VA facilities for needed referrals to a cardiologist, he reports, in hopes of better identifying those at risk for heart attack and stroke. The objective would be improving the decision-making pathway, and thus the quality of care, for military veterans.
As with all such AI-powered algorithms, says Ouyang, it is important to recognize the “limitations and caveats” of the AFib tool. The population with the 12-lead ECGs in the retrospective study may differ from a prospective AFib screening population, for example, and selection bias may occur based on who receives ECGs and care currently. “The algorithm could potentially level the playing field with regard to such biases.”
Leave a comment
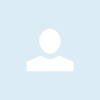
